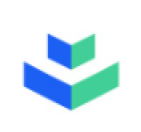
ZIGURAT Institute of Technology
Blog / Leadership & Transformation
Categories
Smart city data is, without a doubt, a hot topic today. But there are many challenges that surround it.
In the age of digital transformation, the emergence of smart cities promises to revolutionise urban living by leveraging advanced technologies to enhance efficiency, sustainability, and quality of life.
However, as the foundation of smart cities lies in data-driven decision-making, there arises a critical challenge: data bias.
The impact of biased data can be far-reaching, leading to inequitable outcomes, reinforcing social disparities, and hindering the potential of smart cities to create inclusive environments.
In this article, we explore the concept of smart cities and delve into the significance of overcoming data bias to ensure equitable decision-making, fostering a future where technology works for everyone.
At its core, a smart city is a connected ecosystem that integrates various technologies to collect and analyse data, with the ultimate aim of improving the quality of life for its residents.
These technologies encompass a wide array of components, such as Internet of Things (IoT) devices, sensors, artificial intelligence (AI), data analytics, and infrastructure networks. By harnessing the power of these interconnected systems, smart cities gather vast amounts of data from diverse sources, ranging from traffic patterns and environmental conditions to energy consumption and citizen feedback.
This data serves as the lifeblood of smart cities, enabling decision-makers to gain valuable insights into urban operations and make informed choices to optimise resources, enhance sustainability, and deliver better public services. By analysing patterns, trends, and correlations within the collected data, city administrators can identify areas for improvement, streamline processes, and devise innovative solutions to address urban challenges effectively.
However, while data holds immense potential, it is vital to recognise that it can be subject to biases that reflect and perpetuate existing societal inequalities.
Objective data analysis has limitations.
“At the heart of data-driven urbanism is a computational understanding of city systems that reduces urban life to logic and calculative rules and procedures, which is underpinned by an instrumental rationality and realist epistemology”.
-Robert Kitchin, Irish geographer
This approach does not capture the complete picture of what is going on, and has the risk of leading to a form of “techno utopianism”, warns Kitchin. Objectivist smart city approaches ignore metaphysical aspects of human life such as politics, ideology, social structure, culture and relationships.
Smart cities face “wicked problems” which need a holistic approach. Wicked problems in the context of smart cities refer to complex and multifaceted challenges that arise when implementing technological solutions to urban issues. These problems are characterised by their ambiguity, interconnectedness, and the lack of a definitive solution. They often involve diverse stakeholders with conflicting interests and require a deep understanding of social, economic, and environmental dynamics. Some examples include equitable access to technology, data privacy and security, citizen engagement, and the ethical use of artificial intelligence. To tackle these challenges, an objective approach is not effective, since it assumes that complex problems can be solved or optimised by monitoring, measuring and computation. This may “paper over” the cracks rather than addressing the deep-rooted structural problems underpinning them.
Utmost faith in technology can be a problem. As Tim Woolliscroft, Senior Design Researcher en TPXimpact and professor of ZIGURAT’s Smart Cities Master, says, the faith in technology may be regarded as a resurgence of the modernist enlightenment vision. The modernist world view assumes data exists independently of the power interests of those who collect it.
Sampling Bias: This type of bias occurs when the data collected is not representative of the entire population within a smart city. It may stem from uneven distribution of sensors or IoT devices, leading to underrepresentation or exclusion of certain areas or demographics. Sampling bias can result in inaccurate insights and decision-making that fails to address the needs and experiences of marginalised communities.
Demographic Bias: Demographic bias occurs when the collected data disproportionately represents certain demographic groups while underrepresenting others. This bias can arise from factors such as digital divides, language barriers, or socioeconomic disparities, leading to skewed understandings of urban dynamics and inequitable resource allocation.
Temporal Bias: Temporal bias refers to the distortion of data due to variations in the time and duration of data collection. For example, if data is predominantly collected during specific hours or seasons, it may overlook important variations or events that occur at different times. This bias can limit the accuracy and reliability of predictive models and decision-making processes in smart cities.
Cultural Bias: Cultural bias arises when data collection methods and algorithms fail to account for cultural nuances, practices, or values of diverse communities. It can result in biased interpretations, recommendations, or policies that do not align with the cultural context of certain population groups, potentially leading to exclusionary outcomes and reduced trust in smart city initiatives.
Algorithmic Bias: Algorithmic bias occurs when the algorithms used to analyze and make decisions based on data perpetuate or amplify existing biases present in the data. This bias can be unintentional, arising from biased training data or flawed algorithm design. Algorithmic bias can lead to discriminatory outcomes in areas such as predictive policing, public service delivery, or resource allocation, further exacerbating social inequalities.
Spatial Bias: Spatial bias refers to the unequal distribution of data collection infrastructure, such as sensors or IoT devices, resulting in biased representations of different geographical areas within a smart city. This bias can impact decision-making related to urban planning, transportation, or environmental management, as certain areas may receive disproportionate attention or resources.
Historical Bias: Historical bias stems from the reliance on past data that reflects historical social, economic, or political inequalities. If historical biases are not addressed, they can be perpetuated in smart city decision-making, reinforcing existing disparities and limiting opportunities for marginalised communities.
Having a more evolutionary and citizen-centric approach is more effective when analysing urban landscapes. This favours a more bottom-up perspective rather than a top-down view. Smart city solutions should require the active participation of all city actors (citizens, consumers, users). Certain tools make use of this approach: journey mapping, prototyping, ethnographic studies and persona development, among others.
“Cities have the capability of providing something for everybody, only because, and only when, they are created by everybody”
-Jane Jacobs, American activist and urban theorist.
Putting forward human-centred design. It is not just technologies that need to be designed, but also the service infrastructure that they fit within. Participatory and human-centred design can help address some of the issues associated with technocratic smart city approaches. One real-world example of human-centred design in smart cities is the implementation of intelligent transportation systems (ITS). ITS aims to improve transportation efficiency, safety, and overall user experience by integrating technology and data-driven solutions. A city with a large population and heavy traffic congestion during peak hours can benefit from data collection and analysis, user research and engagement, intelligent traffic management, multi-modal transportation integration and a process with continuous feedback and improvement.
Implementing Design Labs. They are real-world environments where researchers, businesses, residents, and other stakeholders collaborate to test and co-create innovative solutions for urban challenges. These labs serve as platforms for experimentation, prototyping, and validation of new technologies, services, and policies in a controlled yet realistic setting. They provide an opportunity to assess the viability, usability, and social impact of smart city initiatives, integrating the perspectives and needs of the end-users into the design process. By fostering collaboration and active engagement, living labs facilitate the development of sustainable, human-centred solutions that address urban issues effectively.
A real-world example is the "Smart Dubai Innovation Lab" in the United Arab Emirates, that serves as a collaborative space for government entities, private companies, and individuals to develop and test cutting-edge solutions for urban challenges. The lab focuses on leveraging emerging technologies such as artificial intelligence, blockchain, and Internet of Things (IoT) to transform Dubai into a smarter and more sustainable city.
Understanding the importance of diversity and inclusivity in data collection and analysis. Data serves as the foundation upon which smart city initiatives are built, but if the data itself is biased or excludes certain groups, it can perpetuate existing inequalities. By embracing diversity, both in the collection process and the analysis stage, city managers can ensure that the insights gained reflect the experiences and needs of all residents. Inclusive data collection practices involve actively seeking input from marginalised communities, considering a wide range of socio-demographic factors, and addressing potential biases in data sources. Amsterdam, for example, implemented a project called "Mapping Slums." Recognizing that traditional data sources might not capture the experiences of marginalised communities, the city collaborated with local organisations and residents to collect data about informal settlements and underprivileged neighbourhoods.
Promoting transparency and accountability in data governance processes. This fosters trust among residents and promotes a sense of accountability among those responsible for handling data. Transparent data governance processes involve clearly defining data ownership, outlining privacy safeguards, and establishing protocols for data sharing. By upholding transparency and accountability, cities can build public confidence, protect individual privacy rights, and encourage responsible and ethical use of data for the benefit of all.
Introducing ethical frameworks and guidelines for data collection and use. Ethical frameworks help guide decision-making processes by outlining responsible data practices, including informed consent, data anonymization, and protection of personal information. These guidelines promote transparency, accountability, and the responsible use of data for the benefit of individuals and communities.
Applying Design Thinking and Systems Thinking to urban ecosystems. Design thinking is useful for a smart city manager because it provides a structured and empathetic approach to problem-solving and innovation. By putting the needs and experiences of city residents at the centre of decision-making, design thinking allows managers to understand their challenges, aspirations, and behaviours, leading to more effective and user-centric solutions. It encourages collaboration, creativity, and iterative prototyping, enabling managers to address complex urban issues in a holistic and inclusive manner. Design thinking was applied in the city of Helsinki, Finland, to tackle the challenge of reducing car usage and promoting sustainable transportation. The city implemented a pilot project called "Kutsuplus," a flexible on-demand bus service based on mobile technology.
Systems Thinking enables a holistic understanding of the interconnected components and dynamics within a city. By considering the city as a complex system of interrelated parts, managers can identify the underlying causes and systemic relationships that shape urban challenges. This approach helps in making informed decisions that consider the broader impacts and unintended consequences of interventions. Systems thinking was applied in the city of Curitiba, Brazil, to address urban transportation challenges. The city implemented an integrated transportation system called "Rede Integrada de Transporte" (RIT). This system integrated bus rapid transit (BRT), dedicated bus lanes, and extensive cycling infrastructure.
Implementing robust data validation and verification methods. By ensuring that collected data is accurate, consistent, and trustworthy, cities can make informed decisions based on reliable information. Robust validation and verification processes involve cross-referencing data from multiple sources, applying statistical analyses, and employing quality control measures. These methods help identify and rectify any errors, biases, or inconsistencies in the data, ensuring its suitability for decision-making purposes.
Encouraging collaboration between government, academia, and community stakeholders. By fostering partnerships and open dialogue, cities can leverage diverse expertise, resources, and perspectives to tackle complex urban challenges. Collaboration allows government entities to tap into the knowledge and research capabilities of academia, ensuring evidence-based decision-making and innovative solutions. Engaging community stakeholders ensures that smart city initiatives align with the needs and aspirations of the residents they aim to serve.
The "Equity Atlas" initiative in Portland, Oregon. By analysing and visualising data on various socio-demographic factors, such as income, education, and race, the Equity Atlas highlighted areas of inequity and enabled evidence-based decision-making. This initiative empowered stakeholders to address systemic disparities and allocate resources more equitably, fostering a more inclusive and just smart city environment in Portland.
The "Urban Observatory" initiative in Amsterdam, the Netherlands. This initiative aimed to collect and analyse data on various aspects of urban life, including mobility patterns, air quality, and social dynamics. To address data bias, the Urban Observatory actively engaged citizens through participatory data collection methods. This involved equipping volunteers from diverse backgrounds with mobile sensors to collect data in their neighbourhoods. By involving a broad range of community members, the initiative ensured the inclusion of diverse perspectives and reduced biases in the data.
Graduates of ZIGURAT’s Master's in Global Smart City Management are equipped with the knowledge and skills to critically evaluate data sources, identify biases, and implement strategies to address them. They understand the importance of inclusive data collection practices, ethical frameworks, and transparent data governance. In other words, they are well prepared to deploy smart city data solutions.
With a deep understanding of the social, economic, and environmental complexities of urban environments, these professionals can lead initiatives that prioritise equity, diversity, and inclusivity in data-driven decision-making processes.
In fact, this article was written taken into consideration some of the contents developed by experts Tim Woolliscroft, Lech Rzedzicki, Eduardo Martínez Gil and Emily Griffiths, professors of the programme.
ZIGURAT Institute of Technology